In this paper we present a framework to characterize the pervasiveness and relative importance of bots in various OSNs conversations of three significant global events in 2016. In total, we harvested more than 30 million tweets from the U.S. Presidential Election, the Ukrainian Conflict and Turkish Political Censorship and compared the conversational patterns of bots and humans within each event. The results from this analysis showed that although Twitter participants identified as social bots comprised only 0.28% of all OSN users in this study, they accounted for a significantly large portion of prominent centrality rankings across the three conversations. If you want to know more about this new work, below we provide the abstract to the paper, a selection of figures and tables (including our methodology, some summary information about our data corpus and some of the results). Finally at the bottom of this post we have the full reference and a link to the paper.
Abstract:
The emergence of social bots within online social networks (OSNs) to diffuse information at scale has given rise to many efforts to detect them. While methodologies employed to detect the evolving sophistication of bots continue to improve, much work can be done to characterize the impact of bots on communication networks. In this study, we present a framework to describe the pervasiveness and relative importance of participants recognized as bots in various OSN conversations. Specifically, we harvested over 30 million tweets from three major global events in 2016 (the U.S. Presidential Election, the Ukrainian Conflict and Turkish Political Censorship) and compared the conversational patterns of bots and humans within each event. We further examined the social network structure of each conversation to determine if bots exhibited any particular network influence, while also determining bot participation in key emergent network communities. The results showed that although participants recognized as social bots comprised only 0.28% of all OSN users in this study, they accounted for a significantly large portion of prominent centrality rankings across the three conversations. This includes the identification of individual bots as top-10 influencer nodes out of a total corpus consisting of more than 2.8 million nodes.
Keywords: bots, online social networks, social network analysis.
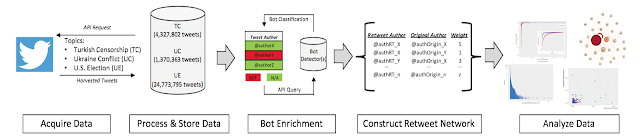 |
Fig. 1. Overall methodology to analyze bot evidence across multiple Twitter OSN conversations. |
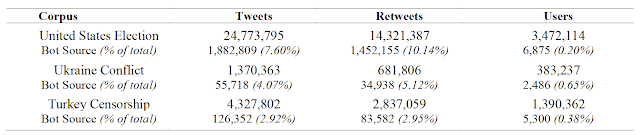 |
Table 1. Harvested Twitter Corpus Overview |
 |
Fig. 5. Correlation of centrality measures for select centrality comparisons: (a) U.S. Election eigenvector versus betweenness analysis, (b) Ukraine Conflict eigenvector versus betweenness analysis and (c)Ukraine Conflict eigenvector versus degree analysis. |
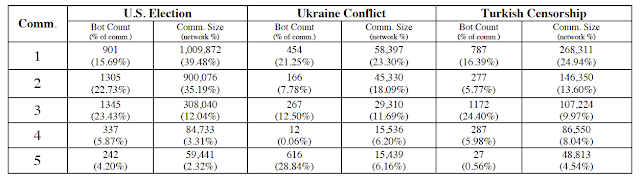 |
Table 2. Bot density of largest emergent communities. |
Full Reference:
Schuchard, R., Crooks, A.T., Stefanidis, A. and Croitoru, A. (2018), Bots in Nets: Empirical Comparative Analysis of Bot Evidence in Social Networks, in Aiello, L.M., Cherifi, C. Cherifi, H., Lambiotte, R., Lió, P. and Rocha, L.M. (eds.),
Volume 2, Proceedings of the 7th International Conference on Complex Networks and Their Applications, Cambridge, United Kingdom, Springer, pp 424-436. (
pdf)